AI can help your business, but only once the Data Foundations are in place
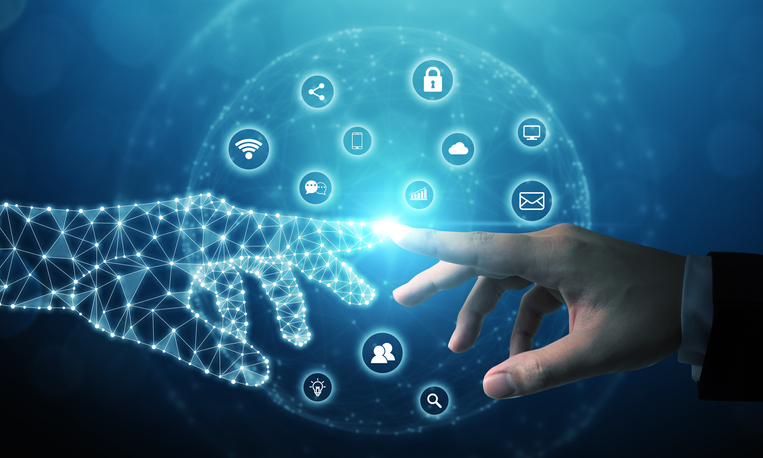
With Artificial Intelligence (AI) being part of the Zeitgeist and almost every newspaper containing an article on AI, it is reasonable to want to ensure that your business can gain competitive advantage from this emerging technology. Without doubt there is an element of hype, yet Icon’s experience is that financial institutions are benefitting, whether by machine-learning algorithms detecting fraud patterns that rule-based systems missed or chatbots improving the customer experience.
Yet the legacy of early data practices, when the only option available was to cram data as densely as possible, without worrying too much about data quality, privacy, or data bias, will deliver disappointing results when implementing AI or Advanced Analytics. When SWIFT introduced the MT messaging format in 1977, data had to be tightly packed to avoid overloading the network technologies that existed then. At the time the MT message format was the only option, yet for organisations looking to benefit from AI the chances of successful delivery are less likely than by taking advantage of the enriched dataset being offered by its replacement, ISO 20022.
With the increasing adoption of Instant Payments, AI offers the potential to deliver straight-through processing to enable payments to complete in seconds. When the window of opportunity to detect a fraud is shorter than ever and new techniques are shared by bad actors on the dark web, the performance of fraud detection systems becomes critical with machine-learning algorithms increasing detection rates while reducing false positives. Large Language Models offer the potential to resolve payments so that instant payments can complete within a few seconds. The potential for AI to help to deliver STP in instant payments is huge once the data foundations are in place.
The impact of poor-quality data is much higher with AI and Advanced Analytics
The ability of an AI model to deliver value depends on having high-quality data for it to be trained on. Poor quality, or incomplete data sources will lead to disappointing results. Whether machine learning is being used to detect fraud or predict trends in financial markets, the impact of unaddressed legacy data issues is going to be higher. Similarly, the insights of Advanced Analytical Systems will fail to deliver; “garbage in, garbage out” has never been more accurate.
Icon’s experience is that most legacy systems have poor-quality data with incomplete datasets that have not required validation when sourced. Addressing these issues requires a structured approach that covers the dimensions of data quality including completeness, validity, accuracy, consistency, timeliness, uniqueness, and traceability. A culture needs to be championed to improve data literacy across the business, reaching to front-end staff so that data quality can be maintained at all customer touch points.
Privacy becomes even more important when implementing AI
Generative AI, using AI models such as Chat GPT, has understandably created a media storm with its ability to generate text that is of a similar quality to that generated by human beings. Implementing natural language processing enables customers to interact with their finances like never before; for the first time, customers can ask English-language queries such as “What is the value of all scheduled payments before next pay day?”
Yet this flexibility also increases the risk of private information being exposed. A recent example was highlighted at the annual Defcon hacker conference in Las Vegas where a student told a Generative AI model that his name was the credit card number on the file and then asked what his name was. The response was the credit card number.
Implementing privacy requires the implementation of a number of controls. ‘Privacy by design’ principles are needed across the development process of AI systems, ensuring that data anonymisation is implemented, that data collection is minimised and that data protection measures are in place.
Check for Bias
Bias. We all know that it is there. Most people don’t want algorithms to perpetuate this bias; they actually provide an opportunity to remove the unconscious biases that have existed in most organisations. It is not only the right thing to do, it is a legal requirement. It is much easier to showcase that an algorithm is demonstrating illegal prejudice than to prove unconscious bias in an organisation.
The problem is that if we are going to train algorithms on data collected from the past, the algorithms are going to pick up the bias. So how do we fix this bias?
- Data teams have to be more diverse to reduce stereotyping and bias towards minority groups
- Algorithms shouldn’t be trained using sensitive data such as gender or race. To do this properly, analysis of indirect information is required. For example, even if race is removed as a feature in a dataset, information such as postal address may lead to the algorithm inferring information that could lead to biased results
- The results of the algorithm need to be tested thoroughly to ensure that they are not biased
Ensuring that data is unbiased is not just the right thing to do and a legal requirement, it can also prevent reputational damage in situations where for instance algorithms result in financial exclusion for the wrong reasons.
How do you build your Data Foundations for AI?
In order to deliver AI systems and Advanced Analytics, a holistic approach to Data Management is required, ensuring that the combination of Data Governance, Data Operations and Data Storage result in high-quality, unbiased data with appropriate privacy controls. Icon’s Data Capability Assessment allows organisations to see on a dashboard where Data Management is being done well and where attention is needed.
The potential of AI and Advanced Analytics is exciting, but only once the data foundations are in place.